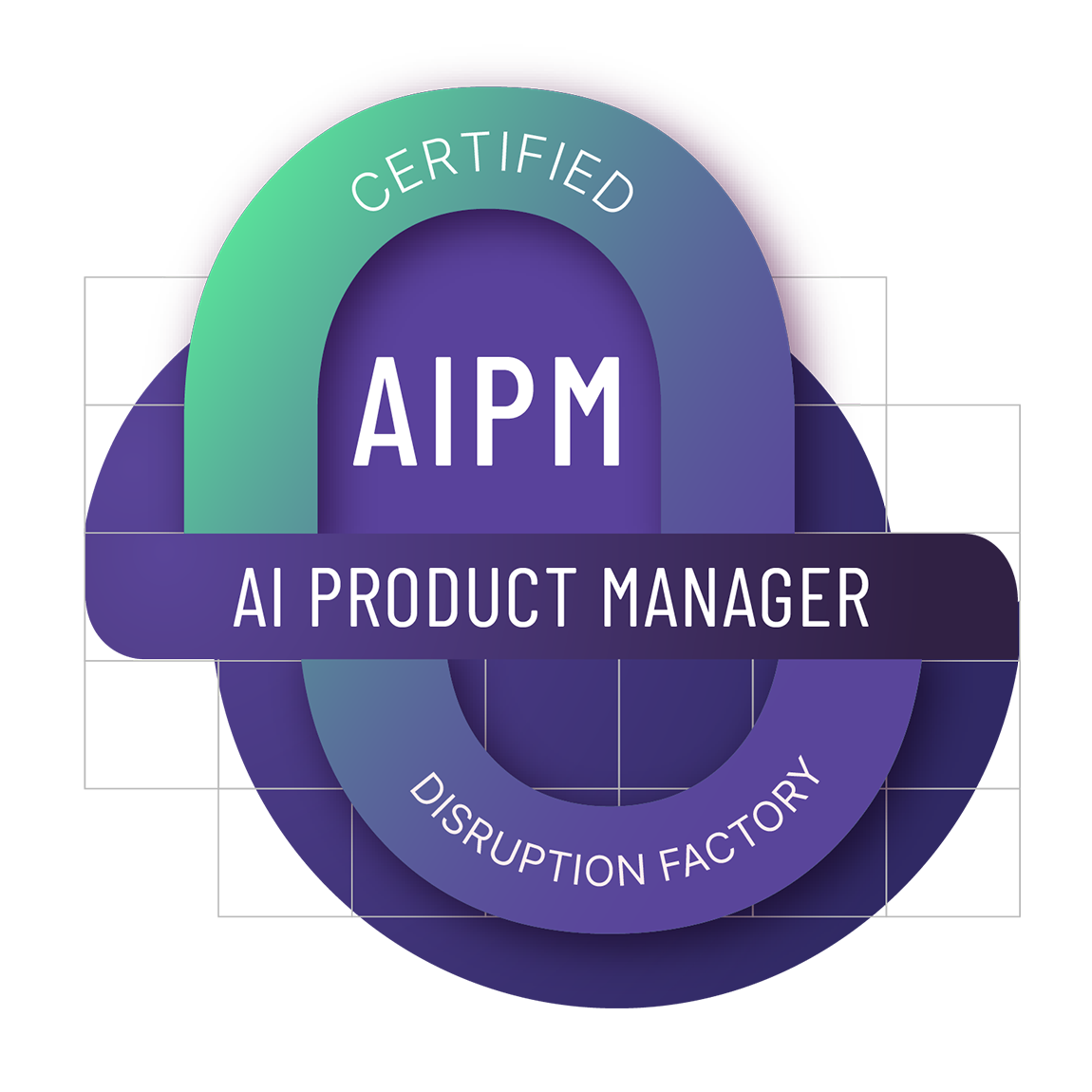
Throughout the exam, you must demonstrate your ability to make strategic decisions based on a deep understanding of artificial intelligence and its application in product management. You are expected to evaluate each scenario critically, avoiding common mistakes such as implementing AI without a clear need, misaligning with business strategy, or neglecting early validation. Every decision you make should reflect a balance between technical feasibility, business impact, and the creation of real value for users.
120min timebox
80% passing score
2 attempts
English or Spanish
Digital Badge through
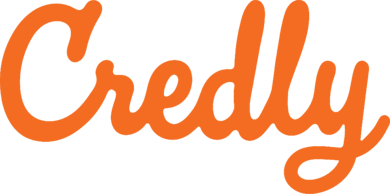
Certification Criteria
Candidates should demonstrate the following competences to be granted the Certified AI Product Manager (AIPM) certification:
1. Fundamentals
1.1. Distinguish the main types of AI (Machine Learning, Deep Learning, Generative AI, Autonomous Agents, among others) and their applications.
An AI Product Manager must possess the critical skill of clearly distinguishing between the main types of artificial intelligence, including Machine Learning, Deep Learning, Generative AI, and Autonomous Agents, as well as deeply understanding their specific applications. This role goes beyond superficial knowledge of available technologies; it requires an in-depth understanding of their particular applications. The role demands strategic comprehension and profound insight, rather than mere superficial familiarity with available technologies.
This competency is fundamental in AI Product Management, enabling informed and strategic decision-making aligned with market expectations and internal team capabilities. A suitable and conscious selection of the type of AI significantly influences product success by optimizing resources, minimizing complexity, and enhancing performance. Conversely, inappropriate or uninformed choices can negatively impact a product's profitability and market acceptance by creating unnecessary complexities, inefficient resource utilization, and operational difficulties.
The importance of this competency lies in its strategic and operational impact. An AI Product Manager skilled in this area ensures not only the technical effectiveness and commercial relevance of a product but also enhances communication with technical teams and stakeholders by establishing clear and realistic expectations. Mastery of this skill ensures technical effectiveness and alignment with commercial objectives, facilitating better collaboration and setting realistic goals in a rapidly evolving technological landscape.
Common Errors
Focusing on “using AI” without analyzing the appropriate type: May lead to wasted resources and features that neither solve the original problem nor generate measurable impact.
Underestimating operational and team barriers: Assuming that any development team can integrate these technologies without anticipating specific requirements may result in delays, cost overruns, and poor user experiences.
Not aligning technology with product strategy: Ignoring the value and purpose of each AI approach can lead to technically impressive features that lack relevance to business objectives and user needs.
1.2. Understanding the Strategic Role of the Product Manager in AI Initiatives
An AI Product Manager plays a fundamental strategic role in artificial intelligence initiatives, serving as a bridge between technology and the organization's business objectives. This role goes beyond simply managing operational or technical tasks; it involves defining a clear AI-based product vision and carefully aligning technological solutions with strategic business needs. By deeply understanding business objectives, the AI Product Manager continuously assesses how specific AI capabilities can strengthen the company’s competitive position and generate tangible value for both customers and the business.
The ability to adopt a strategic role is critical in AI Product Management because it ensures that every technological initiative directly addresses real and relevant business challenges. This skill enables appropriate project prioritization and efficient resource allocation, avoiding unproductive investments or distractions driven by fleeting technological trends. Additionally, a clear understanding of the product's strategic positioning within the market supports informed decision-making on AI integration, critically evaluating the balance between technical complexity, potential value generated, and expected competitive impact.
Effectively exercising this competency positively impacts the organization significantly: it ensures products align with business strategy, strengthens market positioning, and enhances overall competitiveness. It also helps build trust among stakeholders by demonstrating that technological decisions are solidly grounded and aligned with a long-term vision. Conversely, poor management of this competency can result in initiatives disconnected from actual business needs, wasting valuable resources and missing strategic opportunities. This not only negatively impacts the financial performance of the product but could also lead to internal skepticism regarding future AI investments, eroding confidence in the organization's capacity for effective innovation.
Common Errors
Focusing solely on coordinating technical implementation: This may sideline business or product decisions, leading to solutions that lack strategic relevance.
Neglecting cross-functional communication: Failing to align AI initiatives with other teams risks fragmenting efforts and underestimating the complexity of integrating changes into organizational culture.
Focusing exclusively on short-term achievements: Overlooking future growth and maintenance can lead to outdated models, scalability issues, and missed opportunities for continuous innovation.
1.3. Recognizing the Unique Lifecycle of AI Products (Training, Experimentation, Data Dependency)
An AI Product Manager must possess a clear understanding of the unique lifecycle of AI-based products, encompassing specific phases such as model training, iterative experimentation, and critical dependency on quality data. This role extends beyond managing traditional digital product development, requiring deep awareness of how the quality, quantity, and availability of data continuously affect the product's performance and accuracy in production. Therefore, the AI Product Manager must lead strategic initiatives ensuring appropriate selection and curation of data, correct execution of training, and ongoing model validation to maintain effectiveness in real-world operational environments.
Recognizing this particular lifecycle is essential in AI Product Management, as it allows anticipation of specific data-related challenges, such as bias or data drift, which can degrade model accuracy over time. By being aware of these factors from early stages, the AI Product Manager can implement continuous monitoring and optimization strategies, ensuring sustained relevance and effectiveness of the product. Additionally, this skill enhances effective communication with stakeholders, managing their expectations regarding the scope, timelines, and resources required by these initiatives.
The importance of this competency lies in its ability to generate positive impacts, such as optimizing technical and financial resources, enhancing product reliability in production, and maintaining high customer satisfaction through consistent value delivery. Conversely, overlooking or underestimating this unique lifecycle can lead to serious negative consequences, including misdirected investments, unexpected production failures, and rapid product performance deterioration due to inadequate data management or insufficient experimentation. This ultimately results in a loss of stakeholder and customer trust, weakening the organization's competitive position.
Common Errors
Treating AI as an isolated project: Ignoring that models improve with recurrent data and periodic revisions can result in solutions quickly losing effectiveness
Underestimating the complexity of experimentation: Failing to plan adequate resources and methodologies for iterative model development leads to inconsistent outcomes and prolonged time-to-market.
Neglecting data quality and availability: Not prioritizing data management risks training models with insufficient or biased data, significantly reducing product usefulness.
1.4. Adapting Product Management Processes to the Specific Characteristics of AI
An AI Product Manager plays a crucial role in adapting traditional product management methodologies to the unique aspects of AI-driven products. This professional recognizes that the empirical and experimental nature inherent in AI solutions demands more iterative development cycles and greater flexibility throughout the process, given the constant need to validate hypotheses and adjust the product based on real-world interactions with users and production data. This adaptation is not superficial; it represents a deep transformation that ensures technological initiatives remain strategically coherent, even when rapidly redefining based on empirical learnings.
The ability to adapt product management practices to AI-specific requirements directly enhances operational efficiency and strategic effectiveness in developing innovative technological solutions. It enables informed decision-making through frequent experimentation cycles, validation, and iterative learning, thereby optimizing technical and financial resource allocation and reducing the risk of developing features that do not deliver real value. Furthermore, it equips the product team with agility in addressing technical challenges and market shifts, enhancing product resilience against dynamic environmental conditions.
When executed correctly, this competency yields tangible positive outcomes such as sustained value delivery to end users, rapid responsiveness to changing market expectations, and reinforced stakeholder confidence. Conversely, insufficient or improper adaptation can result in rigidity in development plans, complicating the rapid correction of suboptimal decisions and potentially leading to significant investments in solutions that quickly become obsolete. This scenario could cause major delays, frustration among technical and business teams, and a gradual erosion of the organization's competitive advantage within its industry.
Common Errors
Applying methodologies without adaptation: Assuming generic frameworks automatically fit AI environments can lead to roadblocks, rework, and a lack of clarity regarding key objectives or metrics.
Overlooking data management in the process: Ignoring the importance of obtaining, cleaning, and updating data results in inaccurate models, leading to solutions with inconsistent performance or low impact.
Underestimating the need for more agile iteration: Attempting to manage AI initiatives with rigid delivery and validation timelines can frustrate teams and limit opportunities to discover early improvements in models or value propositions.
1.5. Effectively Communicating Technical Implications and Continuous Iteration to Stakeholders
An AI Product Manager holds the critical responsibility of clearly and effectively communicating the technical implications and the necessity of continuous iteration associated with AI-driven products. This role involves more than merely providing superficial technical updates; it requires clearly explaining how technical factors directly influence business strategy, user satisfaction, and commercial outcomes. The ability to clearly convey complex concepts—such as the ongoing need to update and adjust models due to data changes, technical performance management, or correcting inherent errors in AI systems—is essential for fostering shared understanding and strategic alignment among both technical and non-technical stakeholders.
This competency significantly contributes to the success of AI Product Management by fostering transparency and trust among interdisciplinary teams. Effective communication ensures stakeholders properly understand and anticipate the iterative cycles inherent in the development and maintenance of AI solutions, recognizing that absolute perfection from the initial launch is unlikely and that continuous improvement is a core part of the process. This avoids frustration or unrealistic expectations that could lead to internal conflicts and resistance to necessary adjustments stemming from product evolution in real-world environments.
Proper implementation of this competency ensures smooth collaboration between technical and commercial teams, optimizing resource allocation and accelerating the effective adoption of innovative solutions. Conversely, poor communication can cause confusion, mismanaged expectations, and stakeholder resistance, complicating approvals for necessary investments and creating internal friction. In the worst-case scenario, this could lead to significant delays, cost overruns, and loss of confidence in the AI Product Manager and the product itself, negatively affecting overall project perception and ultimately harming the organization's market competitiveness.
Common Errors
Underestimating the importance of transparency: Omitting details about costs, training durations, and testing processes can lead to surprises and tensions among stakeholders.
Not linking technical adjustments to business benefits: Disconnecting product evolution from measurable objectives can result in a lack of commitment and ineffective prioritization.
Handling communication as isolated events: Relying solely on occasional meetings instead of maintaining continuous dialogue fosters misunderstandings about the need for regular iterations and their implications.
2. Product Direction
2.1. Identifying AI-Based Business Opportunities by Connecting Real Needs and Organizational Objectives
An AI Product Manager plays a crucial strategic role in identifying relevant opportunities for AI-based products, ensuring these opportunities are not only technologically feasible but also directly address authentic market problems or specific organizational needs. Their responsibility involves deeply analyzing market demands and internal capabilities, ensuring that each technological initiative is inherently linked to the strategic objectives of the business. This strategic approach prevents the common mistake of adopting artificial intelligence solely due to its technological trendiness, without genuine underlying value or need.
The importance of this competency lies in its direct impact on the operational and strategic effectiveness of AI Product Management. When executed correctly, the Product Manager ensures resources are allocated toward solutions with real potential to create competitive advantages, thus improving the company's market position and strengthening long-term sustainability. Furthermore, the ability to clearly identify and articulate the connection between technology and strategic objectives increases internal confidence and facilitates alignment among technical and business stakeholders, resulting in more effective execution and better product adoption in production.
Conversely, failing to properly exercise this competency can lead to significant negative consequences, such as investing in technologically sophisticated solutions that are misaligned with genuine business needs, thereby wasting resources and efforts. Rushed or superficial implementation of AI initiatives without solid grounding can cause frustration among technical teams, demotivation due to the lack of visible results, and eventually a loss of credibility with stakeholders and customers. Therefore, this competency is essential not only to generate tangible value but also to protect the organization's reputation and competitive position.
Common Errors
Chasing irrelevant use cases: Initiating AI projects merely because they are trendy, without a clear problem to solve, results in products that fail to gain adoption or justify their investment.
Underestimating user and stakeholder feedback: Not actively integrating input from those who use or fund the solution creates gaps between what is offered and what the market or organization truly needs.
Not quantifying potential impact: Lacking metrics and criteria to assess the expected benefits of AI impedes proper prioritization of opportunities, dispersing resources across low-return projects.
2.2. Identifying AI-Based Business Opportunities by Connecting Real Needs and Organizational Objectives
An AI Product Manager plays a critical role in prioritizing opportunities for developing AI-driven products, carefully analyzing each initiative along three key dimensions: potential business impact, technical feasibility, and tangible user value. This role involves accurately evaluating how a specific AI solution can enhance defined business outcomes, simultaneously considering the technical complexity of implementation and how effectively it addresses the genuine needs of end-users. Consequently, the AI Product Manager serves as an essential facilitator, effectively connecting the company's strategic aspirations with realistic technical capabilities and genuine market demands.
This competency significantly contributes to the discipline of AI Product Management, providing a rigorous framework for informed strategic decision-making and preventing the organization from dispersing limited resources on initiatives with minimal potential or technical infeasibility. By focusing solely on projects that robustly meet criteria for impact, feasibility, and user value, the likelihood of commercial success and ongoing product relevance significantly increases. This not only optimizes return on investment (ROI) but also enhances internal alignment and promotes effective collaboration among technical, business, and user experience teams.
When properly exercised, this competency offers organizational benefits such as optimal resource allocation, improved market acceptance of products, and sustained reinforcement of competitive advantage. However, neglecting this prioritization approach entails substantial risks, including fragmented efforts on marginal initiatives, substantial operational cost increases, internal frustration due to stalled or failed projects, and negative stakeholder perceptions arising from unclear outcomes. Over the long term, such scenarios can erode confidence in future AI investments and significantly weaken the organization's strategic and competitive positioning within its industry.
Common Errors
Underestimating development complexity: Pursuing attractive opportunities that present technical or data-related challenges beyond available resources can lead to delays and cost overruns.
Not evaluating differential user value: Selecting projects based solely on internal metrics, without adequately addressing user experience and real-world problems, often results in low-adoption, low-impact solutions.
Neglecting alignment with corporate strategy: AI opportunities that fail to reinforce growth objectives or competitive advantages waste the transformative potential of the technology.
2.3. Balancing Business Value Capture with Solving Relevant User Problems
An AI Product Manager plays a vital strategic role in carefully balancing business value capture with effectively addressing significant end-user problems. This role involves making balanced decisions to ensure that AI-driven solutions deliver financial and strategic benefits to the organization while directly meeting the real and pressing needs of users. This strategic balance positions the Product Manager as a key facilitator who must deeply understand both internal business expectations and the motivations and concrete challenges of the target market.
This competency critically contributes to the field of AI Product Management by ensuring the sustainability and scalability of AI-developed products. By strategically aligning technological initiatives with commercial objectives and user-specific needs, products become not only commercially viable but also positively valued by users, promoting organic and growing adoption. This clear connection between commercial value and user satisfaction fosters robust product creation that remains relevant over time and enhances customer loyalty to the brand.
The importance of this competency lies in its potential to drive positive business outcomes such as increased customer retention rates, sustained satisfaction, and lasting competitive advantage. Conversely, failing to exercise this competency appropriately may expose the organization to significant risks, such as developing technologically sophisticated solutions disconnected from actual user needs, resulting in low adoption or high customer churn. Additionally, focusing solely on immediate financial outcomes can gradually erode users' perceived product value, negatively impacting the company's reputation and its ability to compete effectively in increasingly user-oriented markets.
Common Errors
Focusing exclusively on profitability: Neglecting user experience relevance can lead to solutions perceived as forced or unreliable, negatively affecting adoption and reputation.
Prioritizing usability without a monetization model: Developing attractive features without considering long-term profitability leads to popular but unsustainable solutions, preventing continuous development.
Neglecting mutual feedback between business and users: Failing to map how AI generates business value while satisfying user needs makes it challenging to prioritize improvements and risks product competitiveness.
2.4. Communicating and Aligning AI Product Strategy Across Teams and Stakeholders
An AI Product Manager plays a fundamental role as a key communicator and facilitator of the artificial intelligence-based product strategy across multiple teams and stakeholders within the organization. This role involves clearly defining not only the product’s strategic vision and objectives but also actively ensuring that every team—from technical teams and data scientists to sales and customer service departments—understands how their specific roles contribute to broader organizational goals. This competency is essential due to the complex and multidimensional nature of AI products, requiring all stakeholders to work harmoniously and understand how their efforts impact the product's strategic and commercial value creation.
Properly applying this competency delivers numerous organizational benefits, including enhanced cross-functional collaboration, significantly reduced internal resistance to change, and overall optimized resource allocation by avoiding duplicated or misdirected efforts. Clear and consistent communication of the strategy also enables stakeholders to better anticipate and understand necessary changes throughout the product lifecycle, thereby increasing organizational agility and flexibility. Conversely, poor execution can lead to significant disconnects between teams, causing execution discrepancies, internal friction, and negative perceptions among stakeholders. This can severely impact organizational agility, increase costs, delay progress, and erode the organization's competitive advantage.
Common Errors
Keeping the strategy in silos: Insufficient or delayed sharing of information creates execution discrepancies, delays, and potential conflicts among teams.
Using excessively technical or generic language: Communication disconnected from each audience's context leads to confusion and a lack of engagement.
Neglecting mutual feedback between business and operational teams: Not clearly mapping how AI generates business value while simultaneously satisfying users makes it difficult to prioritize improvements and jeopardizes product competitiveness.
3. Product Discovery
3.1. Defining and Refining Business and User Hypotheses to Ensure Opportunity Alignment with Real Needs
An AI Product Manager plays a crucial role in continuously defining and refining business and user hypotheses to ensure AI-based opportunities align precisely with genuine needs. This professional must deeply engage in strategic analysis and fully understand the genuine challenges facing both end-users and the organization, ensuring proposed solutions are not only technologically innovative but also practically relevant and valuable. This systematic and ongoing validation process helps swiftly filter out weak ideas and reinforces those grounded in genuine problems.
The ability to define and refine these hypotheses is critically important in AI Product Management because it ensures organizational resources are focused on opportunities that have a clear foundation in authentic needs rather than merely being technologically appealing. Correct application of this skill provides tangible organizational benefits, such as efficient resource allocation, improved market performance, and high end-user acceptance due to the solution's direct relevance. Conversely, poor execution of this competency could lead to substantial risks such as investing in products misaligned with user reality or strategic priorities, resulting in low adoption rates, customer dissatisfaction, high costs due to post-launch adjustments, and ultimately erosion of internal and external trust in future AI initiatives.
Common Errors
Assuming AI usefulness without evidence: Launching projects without validating the existence of an actual problem results in irrelevant or poorly adopted solutions.
Defining ambiguous hypotheses: Failing to clearly specify expected business outcomes and user experiences makes it challenging to prioritize opportunities and measure success.
Ignoring iterative hypothesis refinement: Maintaining a rigid approach without adapting as new data emerges can undermine product competitiveness and relevance.
3.2. Designing and Executing Early Validation Experiments (Lightweight PoCs, Quick Tests) to Confirm AI Utility and Feasibility with Minimal Investment
An AI Product Manager plays a fundamental role in conducting early validation experiments, using lightweight Proofs of Concept (PoCs) and quick tests to rapidly verify both the utility and technical feasibility of proposed AI solutions. This professional is responsible for designing agile experiments with minimal investments, focused on testing critical hypotheses regarding the real value the technology could deliver to users and the business. Through these experiments, the AI Product Manager gathers empirical evidence supporting strategic decisions, promptly confirming whether a specific AI approach is viable and effectively addresses concrete needs.
This competency is essential within AI Product Management as it significantly optimizes the use of financial, technical, and human resources, reducing risks associated with large-scale development of unvalidated solutions. Properly applying this competency accelerates organizational learning cycles, swiftly identifying initiatives with potential success and discarding or pivoting those likely to fail before incurring substantial costs. Positive outcomes include more efficient budget allocation, greater strategic agility, and enhanced capacity for innovation, all strengthening the company's competitive position in dynamic markets. Conversely, inadequate execution of these experiments may lead to substantial risks, including significant resource wastage on failed or unnecessarily complex projects, delays in delivering real value, and internal erosion of trust regarding future technological initiatives, seriously compromising the organization's ability to innovate and adapt.
Common Errors
Committing excessive resources in early stages: Beginning with complex developments without preliminary testing can lead to significant cost overruns and delays if the hypothesis proves unviable.
Failing to define clear success criteria: Not clearly specifying what is to be validated by the PoC prevents determining whether results truly justify further investment in AI.
Ignoring feedback from teams and users: By not involving experts and potential customers in early validation, critical insights that could optimize the experiment are missed.
3.3. Evaluating Data Criteria and Initial Metrics to Determine Solution Relevance and Potential Impact
An AI Product Manager holds the essential responsibility of thoroughly evaluating data-related criteria and clearly defining initial metrics, forming an objective basis for determining the relevance and potential impact of AI-driven solutions. This evaluation includes verifying the quality, availability, and relevance of data necessary for developing and operating specific solutions, as well as identifying and establishing key indicators from the outset to accurately measure success and value delivered. Through exercising this competency, the AI Product Manager serves as a strategic gatekeeper, preventing the organization from committing to initiatives that, despite appearing innovative, lack solid grounding in terms of real value creation and measurable outcomes.
The importance of this competency lies in its capacity to strategically focus efforts and resources on initiatives with high potential returns, ensuring a direct link between executed tasks and clearly defined business and user objectives. Properly applied, it enables the organization to minimize risks, reduce unnecessary costs, and accelerate the effective development of high-value, demonstrably high-performance products, thus fostering continuous trust and support from internal and external stakeholders. Conversely, inadequate or superficial evaluation of data criteria and initial metrics can introduce significant operational and strategic risks, such as investments in technically unfeasible solutions or those failing to deliver meaningful results, accumulating costs from late-stage corrections, and a gradual loss of credibility in future AI initiatives within the organization. This lack of rigor can even compromise the company's competitive positioning in highly demanding and dynamic markets.
Common Errors
Ignoring data quality and availability: Overlooking necessary data sources or integrity can lead to inaccurate models and outcomes with limited value.
Underestimating the importance of clear metrics: Failing to define tangible indicators impedes objective evaluation of progress or the effectiveness of the AI solution.
Relying solely on historical data without real validation: Depending exclusively on past patterns without considering changes in user behavior or environmental conditions can result in outdated and fragile decision-making.
3.4. Collaborating with Technical and Design Teams to Rapidly Explore Idea Feasibility and Iteratively Adjust Hypotheses
The AI Product Manager's role in collaborating closely with technical and design teams to rapidly assess idea feasibility is essential. This professional acts as a key facilitator of interdisciplinary collaboration, integrating technical, design, and business perspectives to continuously explore, validate, and refine initial hypotheses for AI-based solutions. Through structured, ongoing direct interaction with technical specialists and designers, the Product Manager can quickly identify technical risks, implementation limitations, and opportunities for improvement early in the development process, ensuring products consistently evolve based on solid evidence and multidisciplinary feedback.
This competency is particularly critical in AI Product Management, significantly contributing to reduced development times and costs, optimized resource allocation, and enhanced final product quality. Properly applying this competency facilitates agile and effective iterations, increasing the likelihood of developing products that closely align with actual user needs and strategic business objectives. Additionally, this cross-functional collaboration strengthens internal trust and cohesion, fostering a culture of continuous learning and improvement. Conversely, inadequately exercising this competency can lead to significant negative outcomes, including late identification of critical technical issues, solutions that are either unfeasible or fail to meet user expectations, and excessive cost accumulation due to late-stage adjustments. Furthermore, poor interdepartmental communication can erode internal collaboration, causing frustration and negatively impacting the organization's capacity to innovate rapidly within a highly competitive environment.
Common Errors
Assuming feasibility as trivial: Underestimating technical complexity or the need for iteration prevents early identification of limitations, leading to costly delays.
Excluding the design team from validation: Relegating design to later phases misses opportunities to explore superior approaches, potentially resulting in poor user experiences.
Subordinating collaboration to rigid processes: Maintaining overly formal structures prevents rapid adjustments and undermines the flexibility necessary for efficient AI solution development.
3.5. Selecting and Prioritizing AI Solutions Based on Impact, Risks, and Cost-Benefit Analysis
The AI Product Manager plays a critical role in selecting and prioritizing AI-based solutions by rigorously and thoughtfully considering three key elements: potential impact, associated risks, and cost-benefit analysis. This role involves conducting a thorough and strategic evaluation that goes beyond the superficial appeal of technological solutions, ensuring that each selected initiative substantially contributes to business objectives, generates clear value for end users, and minimizes potential technical, operational, or financial risks.
By adopting this disciplined approach, the AI Product Manager ensures that the organization invests its limited resources in projects that are clearly profitable, strategically relevant, and technically viable, avoiding deviations toward poorly substantiated solutions or those with low success potential.
This competency is particularly relevant within AI Product Management, as it facilitates optimal resource allocation, improves operational efficiency, and significantly strengthens the company's competitive advantage. When properly applied, this skill allows the organization to achieve rapid value generation, high user satisfaction, and a considerable reduction in operational, financial, and technological risks, thereby increasing trust and support from both internal and external stakeholders for AI-driven technological initiatives.
On the other hand, poor selection and prioritization can lead to significant resource waste, delays in delivering valuable solutions, and high costs resulting from late-stage technical or business errors. Additionally, poorly justified decisions can negatively impact the company’s market reputation and reduce internal motivation due to recurring failures, ultimately limiting the organization’s ability to compete and grow effectively in a dynamic and demanding environment.
Common Errors
Focusing only on novelty: Undervaluing factors such as maintenance, scalability, or actual return on investment leads to “spectacular” but unsustainable solutions.
Ignoring technical and adoption risks: Failing to consider development complexity or user acceptance can delay delivery and damage the product’s reputation.
Applying inconsistent criteria: Changing priorities without clear justification makes it difficult to track results and misaligns teams in execution.
3.6. Developing Functional Prototypes to Demonstrate Technical Feasibility and Business Value
The AI Product Manager plays a fundamental role in leading the creation and validation of functional prototypes that demonstrate the early utility, technical feasibility, and strategic value of AI-driven initiatives.
This professional does not merely identify potential opportunities but actively drives the rapid development of proof-of-concept prototypes, serving as empirical evidence to support future decisions. By presenting these tangible advancements at early stages, the AI Product Manager significantly mitigates uncertainties associated with implementing emerging technologies while fostering transparent and effective communication with both technical and non-technical stakeholders, ensuring their support and commitment to the project.
This competency is crucial within AI Product Management, as it enables the early identification of opportunities and risks associated with AI-based solutions, laying a solid foundation for strategic decision-making. When effectively executed, this skill provides clear benefits, including optimized resource allocation, faster decision-making processes, and continuous strategic alignment of initiatives with business objectives and end-user expectations. Additionally, well-executed prototypes enhance team motivation and commitment by showcasing tangible progress and partial results.
Conversely, poor management of this competency can lead to significant negative consequences, such as misallocation of resources toward unviable or unnecessarily complex solutions, increased costs due to late-stage changes in advanced development phases, loss of stakeholder trust due to the lack of early visible results, perception of inefficiency or inability to materialize strategic technological initiatives within the organization
Common Errors
Creating overly theoretical prototypes: If they do not reflect real-world conditions or use representative data, findings may be distorted, leading to unreliable conclusions.
Minimizing user experience in the prototype: Designing only the technical aspects without considering interaction and perceived value limits validation and may weaken the impact of presentations.
Delaying prototype development until later stages: Waiting to validate the solution when investment is already high prevents early adjustments, increasing costs and prolonging development timelines.
3.7. Validate Assumptions and Gather Early Feedback from Users and Stakeholders to Refine the Proposal
The AI Product Manager plays a key role in ensuring the continuous and early validation of the underlying assumptions in AI-based solution proposals. This validation is achieved through the systematic collection of feedback from both end users and internal stakeholders, enabling agile and well-founded adjustments that significantly improve the product’s alignment with real market needs and the organization’s strategic objectives. In this role, the AI Product Manager not only facilitates feedback collection but also acts as a critical interpreter, translating these findings into actionable changes, ensuring that each product iteration progressively moves closer to fully meeting market expectations and real needs.
This competency is essential within the discipline of AI Product Management because it ensures that decisions are based on empirical evidence rather than unverified assumptions or intuition. When this skill is exercised properly, it significantly minimizes the risks inherent to technological innovation, as it allows for the early detection of misalignments with market expectations or technical and operational limitations, avoiding inefficient investments. Additionally, it facilitates early alignment with key stakeholders, increasing their trust and commitment by making them feel their perspectives are considered from the outset.
On the other hand, poor management of this competency could lead to severe negative consequences, such as launching products disconnected from real user or business needs, resulting in low adoption rates, high operational costs due to late-stage iterations, and significant potential damage to the product and the organization’s reputation in the market.
Common Errors
Biasing validation: Limiting the user sample or failing to ask critical questions can lead to incomplete conclusions that reinforce incorrect assumptions.
Delaying feedback until later stages: Receiving feedback only when the product is already well advanced delays the detection of improvements and increases correction costs.
Ignoring the voice of internal stakeholders: Failing to incorporate perspectives from marketing, sales, or customer service risks launching solutions that do not integrate with the rest of the organization or lack key support.
3.8. Iterate Quickly to Optimize Prototypes, Incorporating Continuous Learnings to Prepare the Product for Later Stages
The AI Product Manager plays a fundamental role in the agile and efficient iteration of prototypes developed for AI-based solutions, actively incorporating learnings derived from continuous feedback and empirical data. Their role is not limited to managing traditional development processes; it involves a dynamic and highly responsive responsibility that requires quickly adapting to emerging results and adjusting the product’s direction in real time. Through frequent agile cycles, the AI Product Manager progressively refines prototypes, ensuring that each iteration moves closer to a viable, robust solution aligned with strategic objectives and user expectations.
This competency is essential in the discipline of AI Product Management because it significantly reduces the risks inherent to technological innovation. It allows for rapid detection of errors, technical issues, or deviations from initial expectations, enabling timely corrections that prevent high costs and significant delays in later stages. When executed correctly, this competency accelerates the development process, enhances the quality and relevance of proposed solutions, and builds greater trust among internal and external stakeholders, who observe a transparent, well-grounded, and tangible evolution of the product.
Conversely, slow or ineffective iteration can lead to serious negative consequences, such as the accumulation of undetected technical problems, resource waste, and substantial delays in time-to-market. This can erode the confidence of both internal stakeholders and end users, negatively impacting product adoption and weakening the organization’s competitive position in its market.
Common Errors
Extending each improvement cycle excessively: Prolonging iterations without clear goals dilutes agility and delays the collection of valuable insights.
Ignoring unfavorable findings: Dismissing results that suggest deep product changes prevents the solution from reaching an optimal level.
Working in isolation from the team: Keeping iteration separate from a collaborative approach involving design, development, and business limits the overall vision and slows down the adoption of necessary adjustments.
4. Product Delivery
4.1. Plan the Training and Evolution of Models, from Prototypes to Production Environments
An AI Product Manager plays a critical role in strategically leading the training and continuous evolution of AI models, ensuring that these processes are seamless and aligned with the business’s overall strategy. This responsibility involves overseeing all phases of the model development cycle, from initial selection and data preparation, through iterative training and continuous validation, to effective deployment in operational environments. Beyond the purely technical aspect, the AI Product Manager ensures that each training stage is supported by clear criteria and relevant metrics, maintaining a holistic vision of how these processes deliver tangible value to users and impact the organization’s defined objectives.
The ability to effectively manage this training and evolution cycle is fundamental in AI Product Management, as it directly affects the final quality of the product and its ability to quickly adapt to market changes or shifts in data patterns. When executed correctly, this competency significantly optimizes technical and financial investments, reduces operational and technical risks, and greatly enhances product stability and performance in production.
Conversely, poor or unstrategic management of model training can lead to serious negative consequences, such as loss of product quality, high costs due to recurring errors, and reduced return on investment due to constant technical rework. Additionally, it can quickly erode stakeholder and user trust, affecting product adoption and limiting the company’s ability to fully capitalize on AI’s strategic potential.
Common Errors
Underestimating data requirements for each phase: Failing to recognize the magnitude and quality of data needed can stall model maturity and increase costs.
Failing to anticipate retraining and updates: Assuming that a model will remain relevant without periodic oversight leads to outdated results and low adoption.
Treating prototypes as final solutions: Deploying models without a transition or scaling plan hinders standardization, quality control, and user satisfaction in production.
4.2. Identify Technical and Operational Requirements to Scale AI Solutions
An AI Product Manager plays a crucial role in the early and strategic identification of the technical and operational requirements necessary to successfully scale AI-based solutions. This involves a comprehensive understanding of technological infrastructure, specific storage and processing needs for large volumes of data, as well as the clear definition of processes for continuous maintenance and periodic updates of AI models in production. Additionally, this professional is responsible for effectively coordinating efforts across interdisciplinary teams, including engineering, data science, operations, and business, ensuring that the AI solution scales harmoniously and aligns with the organization's strategic objectives.
This competency is key in the discipline of AI Product Management because it ensures that the solution can adapt quickly to increasing data and user volumes, preventing technical or operational limitations that could compromise the product’s growth or stability. When properly executed, this skill enables the AI Product Manager to respond quickly and proactively to growing market demands, thereby maximizing user satisfaction, ensuring the product’s technical robustness, and protecting the profitability of the technology investment.
Conversely, failing to identify these requirements early can lead to significant negative consequences, such as recurring operational failures, loss of model performance due to infrastructure saturation or insufficient data, and high costs associated with late-stage adjustments and corrections. Ultimately, these mistakes could erode internal trust, increase frustration among involved teams, and jeopardize the product’s long-term continuity, competitiveness, and relevance.
Common Errors
Assuming the current infrastructure is sufficient: Relying on generic technological resources without anticipating processing and storage needs can slow down or prevent product expansion.
Neglecting team orchestration: Failing to coordinate developers, data scientists, and operations leads to poorly integrated implementations, delays, and inconsistent service quality.
Not planning monitoring and support procedures: Without setting up tracking routines and early alert mechanisms, performance or quality issues may be detected too late, affecting the product’s reliability and reputation.
4.3. Align Backlog and Roadmap to Integrate AI Features into the Core Product
An AI Product Manager has the strategic responsibility of ensuring that both the backlog and roadmap are perfectly aligned to effectively integrate AI-powered functionalities into the core product. This task requires proactively managing prioritization, ensuring that AI initiatives are not treated as isolated projects but as organic enhancements that reinforce predefined strategic objectives. In this role, the AI Product Manager acts as the connecting link between technological vision and business priorities, clearly translating AI capabilities into concrete, aligned tasks that are understood and supported by both technical teams and business stakeholders.
The importance of this competency lies in its ability to ensure strategic and operational consistency between AI functionalities and traditional product features, preventing fragmented and potentially counterproductive efforts. When executed correctly, it delivers clear benefits, such as better resource utilization, an agile and structured release of new functionalities, and a smoother, more relevant user experience. Additionally, a well-aligned backlog and roadmap enhance internal and external communication, increase confidence in the product, and strengthen competitive advantage by demonstrating constant and effectively integrated innovation.
Conversely, poor execution of this competency can lead to significant risks, such as resource dispersion on disconnected initiatives, lack of long-term clarity, and internal conflicts over product direction. These issues can result in delays, increased costs, organizational frustration, and ultimately, a significant loss of competitiveness and relevance compared to competitors who successfully integrate AI in a more agile and strategic manner.
Common Errors
Treating AI features as isolated initiatives: Disconnecting AI enhancements from the main roadmap creates work overload and reduces coherence in the value proposition.
Failing to prioritize integration with existing releases: Indefinitely postponing AI feature insertion in key launches can delay market validation and weaken momentum.
Ignoring feedback and adoption metrics: Failing to monitor how users interact with new AI functionalities misses the opportunity to optimize the roadmap and improve iteratively.
4.4. Define Strategies to Mitigate Technical Risks (Performance, Costs, Availability)
An AI Product Manager plays a key role in proactively identifying potential technical risks inherent to AI-based solutions, particularly those related to product performance, associated costs, and continuous service availability. Their responsibilities include defining and implementing specific strategies to mitigate these risks from early stages, analyzing different possible scenarios, establishing clear contingency plans, and optimizing the technical infrastructure to adequately support operational demands. This meticulous approach not only ensures consistent and predictable product performance but also facilitates effective budget management, allowing for cost control and minimizing financial deviations that could affect project profitability.
The ability to anticipate and effectively manage these technical risks is especially critical in AI Product Management due to the dynamic and complex nature of AI-based products, which often face challenges such as data drift, fluctuations in computational resource demand, and unexpected model performance variations. By properly implementing risk mitigation strategies, the AI Product Manager significantly strengthens stakeholder confidence, enhances user experience through high availability and stable product performance, and optimizes budget allocation, preventing additional costs from unforeseen contingencies.
Conversely, insufficient or incorrect risk management can lead to serious negative consequences, such as frequent service disruptions, excessive expenses from ad-hoc or urgent fixes, deterioration of the product's reliability perception in the market, and even customer losses to competitors with more robust and stable products.
Common Errors
Underestimating demand spikes: Ignoring variability in usage or the need for scaling can cause service disruptions and poor user experiences.
Neglecting cost management: Failing to design monitoring and optimization processes can lead to excessive expenses and inefficiencies that hinder the sustainability of the AI solution.
Not implementing redundancy plans: Focusing only on an ideal architecture without considering potential failures prevents timely responses to incidents, undermining confidence in the product.
4.5. Implement Advanced Prompt Engineering and Testing Practices for Large Language Models (LLMs)
An AI Product Manager plays a crucial role in implementing advanced prompt engineering practices and rigorous testing strategies for Large Language Models (LLMs). This role involves not only defining how interactions with the model are structured from a technical perspective but also ensuring that these interactions fully align with the organization's strategic objectives and user expectations. By designing prompts meticulously, the Product Manager optimizes the model’s responsiveness, significantly improving the accuracy, coherence, and practical utility of the results obtained.
This competency is fundamental in AI Product Management, as the quality of interactions with LLMs depends directly on well-designed prompts and continuous validation through structured testing. Effective execution enables the delivery of consistent and enriched user experiences, increasing trust in the technology and facilitating widespread adoption. Additionally, these practices ensure proactive risk management, enabling early detection of biases, errors, or inconsistencies that could damage the product’s public perception.
Conversely, failing to implement these practices properly introduces significant risks, such as erratic or inaccurate responses, user dissatisfaction and distrust, higher operational costs due to late-stage corrections, and, ultimately, a negative market perception of the product. These issues could severely impact product adoption, reduce competitiveness against better-prepared alternatives, and gradually erode the organization’s strategic advantage in the effective and reliable application of AI.
Common Errors
Failing to systematize prompt validation: Relying on isolated or random tests can lead to inconsistent and confusing responses, negatively impacting the perceived quality of the product.
Ignoring the inherent complexity of LLMs: Underestimating the need for robust and repeatable test cases results in unpredictable outputs, reducing reliability in real-world use cases.
Overlooking model evolution: Not periodically re-evaluating and recalibrating prompts as data or model configurations change limits continuous improvement and compromises the product’s long-term relevance.
4.6. Design and Manage the Data Pipeline for RAG (Retrieval-Augmented Generation), Ensuring Relevance, Efficiency, and Ethical/Legal Compliance
An AI Product Manager has the strategic responsibility of designing and overseeing the data pipeline specifically for Retrieval-Augmented Generation (RAG) solutions. This role involves carefully structuring the process of data collection, organization, and retrieval to ensure that the model always accesses relevant, up-to-date, and efficiently delivered content for users. Additionally, the AI Product Manager must ensure that every stage of the pipeline strictly adheres to ethical and legal standards, particularly regarding privacy, data consent, and transparency, in order to protect the organization's reputation and build trust among both end users and internal and external stakeholders.
The strategic importance of this competency lies in its ability to substantially improve the accuracy, relevance, and practical utility of AI-generated responses, thereby strengthening user satisfaction and retention. Proper management of the RAG pipeline also optimizes the product's operational performance by eliminating redundant and costly processes, increasing technical efficiency, and reducing latency in user-model interactions.
Conversely, a poorly implemented or insufficiently regulated pipeline can introduce significant risks, such as the frequent generation of irrelevant or inaccurate responses, leading to user frustration and erosion of trust. Even more critically, negligent handling of ethical and legal aspects could expose the organization to severe regulatory issues or reputational conflicts due to privacy violations or lack of transparency. As a result, proper execution of this competency is essential for the long-term sustainability and success of AI solutions in a competitive and regulated market.
Common Errors
Neglecting data quality and curation: Allowing outdated or unreliable data into the pipeline directly weakens results and increases bias in model generation.
Ignoring legal compliance and data governance: Failing to implement access controls, anonymization, or secure storage can lead to sanctions, reputational damage, and loss of credibility.
Failing to adapt the pipeline to model evolution: Keeping static structures and processes prevents the integration of new data sources or adjustments to changing demands, hindering the continuous improvement of the RAG solution.
4.7. Manage the Transition to Production and Coordinate Internal/External Adoption of New Capabilities
An AI Product Manager has the strategic responsibility of effectively managing the transition to production for AI-based solutions, ensuring that both internal teams and external users are fully prepared to adopt these new technological capabilities. This role involves not only overseeing the technical aspects of the launch but also paying close attention to change management, including comprehensive internal and external communication plans, targeted training for end users and technical teams, and robust support structures during the initial deployment stages. Through this approach, the AI Product Manager ensures that all relevant stakeholders clearly understand the value, functionality, and benefits of the solution, minimizing resistance and enabling seamless integration into standard operational workflows.
This competency is fundamental in AI Product Management as it directly contributes to the successful and rapid acceptance of technological solutions in the market while also mitigating potential adoption risks. When executed properly, this competency enhances the product’s positive perception, accelerates return on investment, and increases overall satisfaction by minimizing uncertainty and difficulties during the transition.
Conversely, poor planning or weak coordination in this critical phase can result in serious negative consequences, such as internal resistance to change, user frustration due to lack of clarity or inadequate support, and a general decline in trust toward both the solution and the organization. Ultimately, these risks may lead to low product adoption, increased costs from unforeseen issues, and potential loss of credibility in future technological initiatives.
Common Errors
Launching without an enablement plan: Underestimating the need for training stakeholders and establishing feedback channels can lead to confusion and resistance to change.
Underestimating the complexity of the production environment: Ignoring the differences between test and production environments (e.g., integrations, security, scalability) can cause failures that harm the end-user experience.
Disconnecting external adoption from internal readiness: Failing to involve internal teams and key users from the start makes it harder to resolve incidents, optimize the solution, and effectively evangelize the value AI provides.
4.8. Collaborate with Development, Data, and DevOps Teams to Streamline Workflow
An AI Product Manager plays a key role as an active facilitator, ensuring effective collaboration among development, data, and DevOps teams to optimize workflow throughout the entire lifecycle of AI-driven products. This role involves maintaining continuous and seamless communication between these technical teams, proactively identifying bottlenecks, aligning technical and business expectations, and promoting agile processes that enable the rapid integration and deployment of solutions. By maintaining constant alignment, the AI Product Manager ensures that AI initiatives are executed with greater speed, efficiency, and quality, strengthening the organization's ability to adapt quickly to market changes.
The importance of this competency lies in its ability to significantly improve internal productivity, reduce development time, and accelerate the delivery of real value to users and the organization. By fostering effective cooperation, technical and operational bottlenecks are minimized, ensuring that each development phase flows efficiently, from initial definition and prototyping to final implementation and continuous operation.
Proper management of this competency promotes high satisfaction and cohesion within involved teams, as well as greater trust and support from stakeholders, thanks to frequent and transparent deliveries. Conversely, poor collaboration can lead to recurrent delays, operational inefficiencies, increased costs, and frustration among technical teams and stakeholders, ultimately deteriorating product quality perception, hindering market adoption, and weakening the organization’s strategic position against competitors.
Common Errors
Keeping communication siloed: Failing to promote visibility and synchronization between development, data, and DevOps leads to delays, implementation errors, and duplicated efforts.
Neglecting automation tools and processes: Ignoring the benefits of CI/CD or infrastructure-as-code management results in manual efforts and increased risk of errors or inconsistencies in AI deployment.
Failing to set clear priorities: A backlog without well-defined criteria can cause confusion about which tasks are critical for delivery, slowing progress and impacting the final product’s quality.
5. Continuous Monitoring and Optimization
5.1. Define Key Metrics (Technical and Business) to Monitor AI Performance
An AI Product Manager plays a strategic role in the definition and rigorous tracking of both technical and business metrics, ensuring a comprehensive evaluation of the performance of AI-driven solutions. On the technical side, they must define metrics such as latency, model accuracy, response times, and operational stability, which are crucial for maintaining optimal production performance. At the same time, they must establish business-related indicators, such as return on investment (ROI), adoption rates, and customer satisfaction, which quantify the solution’s contribution to strategic objectives and organizational growth. This balance positions the AI Product Manager as the primary overseer of the real value AI delivers to the organization and its users.
The importance of this competency lies in its ability to provide a clear, objective, and evidence-based view of the impact and performance of AI solutions. When properly implemented, this competency enables early detection of deviations, supports strategic decision-making, and strengthens organizational trust by providing constant transparency on results.
Conversely, poor execution of this competency introduces significant risks, such as failing to identify technical or business issues early, which can lead to low perceived value by users, resource wastage, and misalignment with strategic goals. In the long run, a lack of clear metrics could result in inability to justify further AI investments, decreased internal motivation for tech initiatives, and weakened competitive positioning against more agile and results-driven competitors.
Common Errors
Not linking technical metrics to business outcomes: Focusing solely on model performance KPIs without connecting them to financial or strategic impact makes it difficult to assess AI’s true value.
Defining too many indicators: Overwhelming teams with irrelevant or excessive metrics dilutes focus and reduces the ability to respond quickly to the most critical signals.
Underestimating the evolution of metrics: Failing to adapt KPIs as the product, user base, or market changes prevents effective and up-to-date performance monitoring.
5.2. Detect and Manage Issues Like Data Drift, Model Degradation, and Prediction Errors
An AI Product Manager plays a critical strategic role in implementing effective systems to detect and manage operational challenges in AI-driven products, such as data drift, model degradation in production, and prediction errors. Their responsibilities include continuous monitoring of both technical performance and the quality of incoming and outgoing data, ensuring early detection of deviations or drops in model accuracy that could negatively impact user experience or business objectives. Additionally, the AI Product Manager must coordinate cross-functional efforts to analyze these issues, determine their root cause, and design agile responses to quickly restore the product's expected performance.
The importance of this competency lies in its ability to safeguard both the quality of user experience and the strategic business outcomes over time. Proper execution of this skill enables timely interventions that maintain model reliability and strengthen trust among internal stakeholders and end users. By effectively managing these technical challenges, the AI Product Manager helps avoid high costs associated with prolonged incidents, prevents customer churn due to dissatisfaction, and preserves the product’s positive market reputation.
Conversely, poor detection and management of these issues can lead to serious negative consequences, such as rapid AI model obsolescence, sustained declines in customer satisfaction, significant financial losses from operational disruptions, and irreversible erosion of trust in future technological investments.
Common Errors
Blindly trusting initial metrics: Assuming that accuracy or performance indicators will remain stable without continuous oversight can result in unnoticed quality deterioration.
Failing to establish a feedback process: Lacking a formal mechanism to log and analyze prediction failures or missing update channels for model improvements limits continuous enhancement.
Underestimating the impact of data drift: Ignoring how patterns evolve over time prevents agile responses to new trends and user behaviors, leading to model obsolescence.
5.3. Ensure Sustained User Value Delivery Through Iterative Model Improvements (Re-Training, Prompt Refinement, Dataset Updates, etc.)
An AI Product Manager plays a key role in ensuring that AI-driven products deliver sustained value to users through a continuous and systematic process of iterative improvements. This role involves continuously monitoring the effectiveness and accuracy of the model in production, identifying the need for strategic adjustments, such as periodic re-training, prompt refinement, or dataset updates. Through these continuous improvement cycles, the AI Product Manager ensures that the product not only maintains its technological relevance but also remains consistently aligned with the evolving needs and expectations of both users and the business.
The importance of this competency lies in its ability to extend the product’s value in dynamic environments, where both data and user behaviors constantly evolve. When properly executed, this competency leads to highly positive outcomes, such as greater user satisfaction, sustained increases in adoption and retention rates, and a strengthened competitive advantage due to the product’s continuous adaptability and relevance in the market.
Conversely, neglecting this competency can introduce significant risks, such as progressive model degradation, a decline in perceived quality, and gradual market share loss to more up-to-date and user-centric solutions. Additionally, failing to respond quickly to changes can result in high costs from late-stage corrections and growing internal distrust toward future AI-based initiatives.
Common Errors
Adopting a “deliver and forget” mindset: Failing to establish a periodic re-training or refinement process leads to gradual performance degradation, negatively impacting user satisfaction.
Neglecting feedback after each adjustment: Ignoring user or operational team responses after a model change limits the ability to correct errors and optimize results.
Failing to assess the relevance of data and prompts: Relying on outdated datasets or prompts prevents the system from evolving with market conditions, resulting in inaccurate predictions and loss of customer value.
5.4. Coordinate the Communication of AI Solutions’ Results and Impact to Stakeholders
An AI Product Manager plays a fundamental role in effectively coordinating communication about the results and tangible impact of AI-based solutions to all relevant stakeholders. This function goes beyond simply reporting metrics or technical progress—it requires clearly explaining how the implemented solutions specifically contribute to the organization's strategic and business objectives, as well as the perceived value for end users. Through strategic and proactive communication, the AI Product Manager ensures that various stakeholders—from executives to technical and commercial teams—fully understand the product’s real impact, creating clear alignment between initial expectations and actual results in production.
The critical importance of this competency lies in its ability to strengthen both internal and external trust in AI-driven initiatives. Effective communication promotes recognition of AI’s real value, increasing support for future investments and enabling continuous product evolution. When properly executed, this competency leads to clear positive outcomes, such as greater credibility among involved teams, increased organizational motivation, and better strategic alignment for future technological investments.
Conversely, insufficient or unclear communication can cause significant negative consequences, including deterioration in the perceived effectiveness of the product, loss of internal support for future initiatives, stakeholder distrust regarding AI’s real return on investment, and difficulties in justifying future strategic tech projects. These issues can deeply impact the organization’s ability to innovate and adapt in a competitive environment that demands agility and continuous transparency.
Common Errors
Presenting only isolated data: Focusing on technical metrics or results without contextualizing their relevance to the business or user experience prevents stakeholders from understanding AI’s true value.
Omitting challenges and areas for improvement: Downplaying challenges or unresolved learnings creates unrealistic expectations and complicates planning for future improvements.
Lacking a coherent narrative: Communicating efforts and achievements inconsistently, without a clear storyline, can confuse stakeholders or diminish the perceived impact of AI solutions within the organization.